Feeding Study Round 2
using motion capture and forces to determine fork approach and trajectory
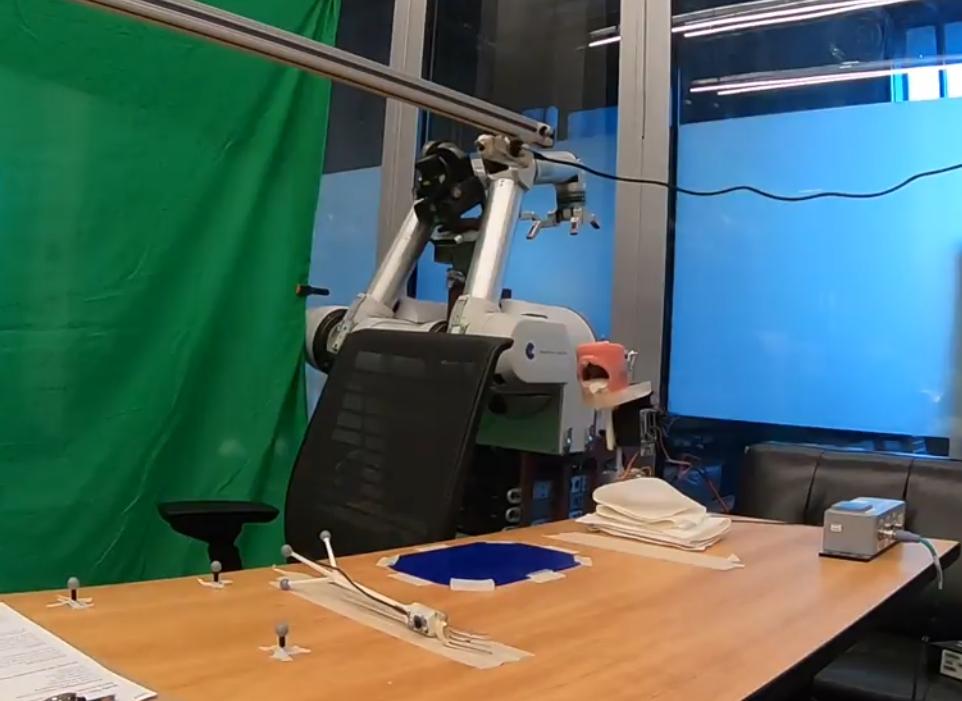
Currently ADA, the Assistive Dextrous Arm, can pick up foods in a highly constrained and contained lab environment. It’s limited to foods that are precut and come in defined shapes like cantaloupe and hard-boiled eggs cut into pieces. Until its repertoire of foods is broadened, ADA can’t be placed into the home for everyday use.
I designed a research study to help fix that. This study’s purpose was to create and analyze a new dataset of foods closer to the diet of one of our end users. We added 13 new foods from one of our co-designer’s weekly diet and had participants pick up bites of food and feed it to our mechanical mouth while we captured the details with a motion capture setup. These foods included precut items such as bagel and sandwiches cut into bite-size pieces as well as foods not traditionally portioned by bites, such as jello, mashed potato, and noodles.
I helped clean and analyze the data so that we can we can run both this new data and data taken from a previous study done in 2018 through an action schema and classify the each action in the bite aquisition. With a large set of generalizable actions we will be one step closer to picking up all different kinds of food. This data set was eventually published as a dataset on Harvard Dataverse Link here.
The results of this study were submitted and accepted to the 2023 Conference on Robot Learning (CoRL).